Using Next Best Merchant to make intelligent recommendations
Using Next Best Merchant to make intelligent recommendations
OCBC Bank constantly runs marketing promotions with external merchants such as restaurants and fashion outlets. At any point, we may be running activities with hundreds of partners.
Understanding which partner offers should be shown to our customers is complex, considering that we have millions of customers. Historically, this decision was made by product managers by developing business hypotheses on which partners might be relevant for different segments. However, this approach was not scalable and delivered sub-optimal returns.
That’s why our Group Data Office developed the Next Best Merchant (NBM) platform to solve this problem and to ensure that our customers receive partner offers that are most likely to meet their needs.
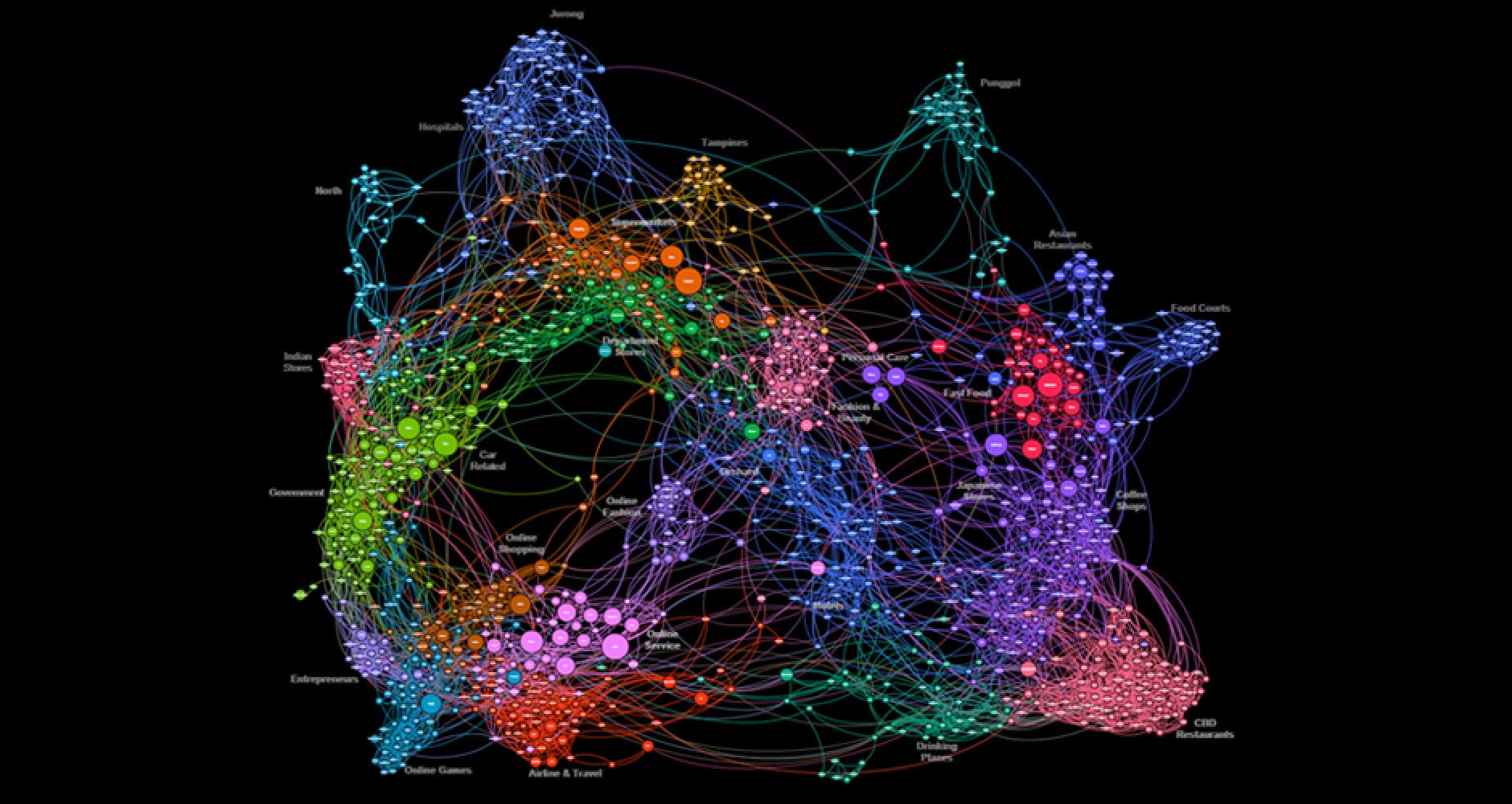
“Next Best Merchant allows our customers to discover relevant new merchants that they will love – but just don’t know it yet.”
— Andrea Pisoni, Head of AI Lab, Group Data Office
Traditionally, recommender systems (models which aim to make the best possible recommendations) fall into one of two categories:
- Collaborative filtering: These models use the interaction between users and products to determine a recommendation. Interestingly, collaborative filtering algorithms do not need to know anything about the users or the products to offer a recommendation. Therefore, they are often used by businesses that do not have a rich set of information about their customers. NBM could have been built using collaborative filtering by leveraging OCBC customer’s historical transaction data.
- Content-based: Contrary to collaborative filtering models, content-based recommender systems leverage users and product features to make recommendations. Since content-based systems don’t leverage user-product interactions, they are mostly used when such interactions are relatively rare. For example, a real estate portal recommending the perfect house to its users would likely produce a better recommendation leveraging user demographics and income data, rather than their previous real estate transactions, as most people only purchase real estate infrequently during their lifetime.
As the two approaches complement each other, many of the newer recommender system algorithms (Fast Factorisation Machines, Neural Collaborative Filtering and many others) have started offering the option to combine the two methods using both user and product data, as well as their interactions.
Thanks to our extremely rich data set, we are able to leverage this hybrid approach to achieve the best possible results. Our model generates recommendations by leveraging customer demographics, transaction history, behavioural patterns and features such as the location of the merchants and the customer’s usual “spend hot-spots”.
Like all our models, NBM uses our MLOps (machine learning operations) platform which allows for it to be deployed as a RESTful (representational state transfer) Merchant Recommendation Service. When a business team submits a campaign request for a new merchant, the model is triggered and fine-tuned to automatically generate the best leads for a given campaign. Through this automated approach, NBM powers hundreds of marketing campaigns each year with minimum manual maintenance and overhead costs.
Customer responses show that the NBM platform works. Results show a typical uptake of three times compared to historical targeting techniques and went as high as ten times for some merchant types.